Artificial intelligence (AI) is now an important part of our daily lives. It is present in the industrial processes of designing the goods we consume, in the online applications we use, in the smartphones we interact with on a daily basis, and even in our homes through voice-controlled home automation systems. Indeed, AI models are becoming increasingly useful in almost every sector, due to their ability to solve various tasks simultaneously or to process huge amounts of data with high accuracy at minimal cost.
Today, AI is playing an increasingly important role in healthcare. Integrating AI into the healthcare ecosystem offers many benefits, such as automating tasks and analyzing large patient datasets. This not only provides better, faster and cheaper healthcare, but also improves care operations management systems.
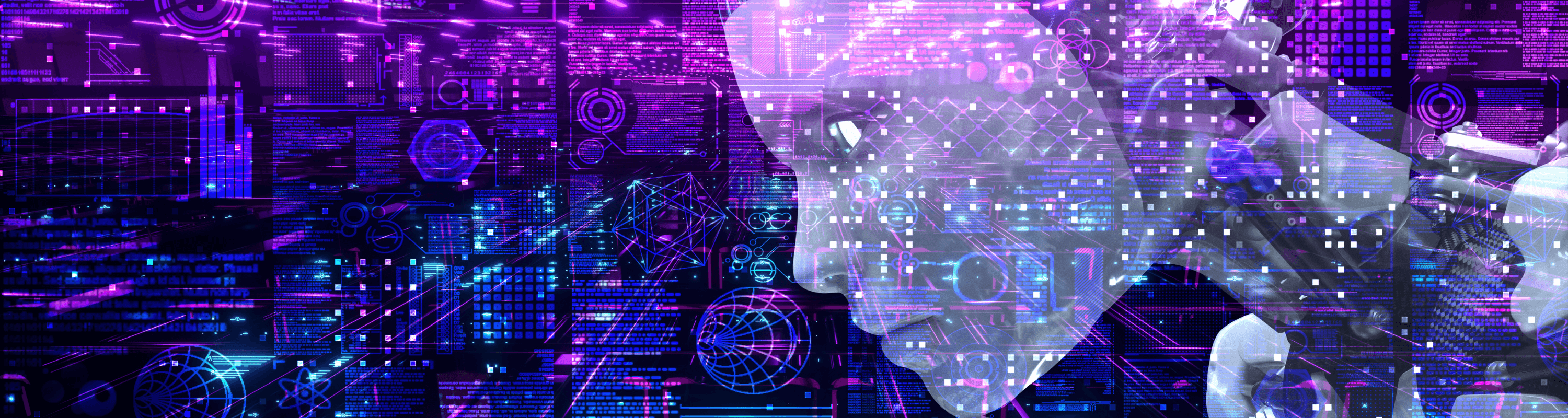
In concrete terms, what is AI and how does it work?
Very simply, an AI model is a tool or algorithm that, given a set of input data, can process new data and arrive at a decision without requiring human intervention in the decision-making process. To go into more detail, artificial intelligence is a constellation of different technologies that work together to enable machines to perceive, understand, act and learn at levels of intelligence comparable to humans. This probably explains why there are so many definitions of artificial intelligence: AI is not limited to one technology.
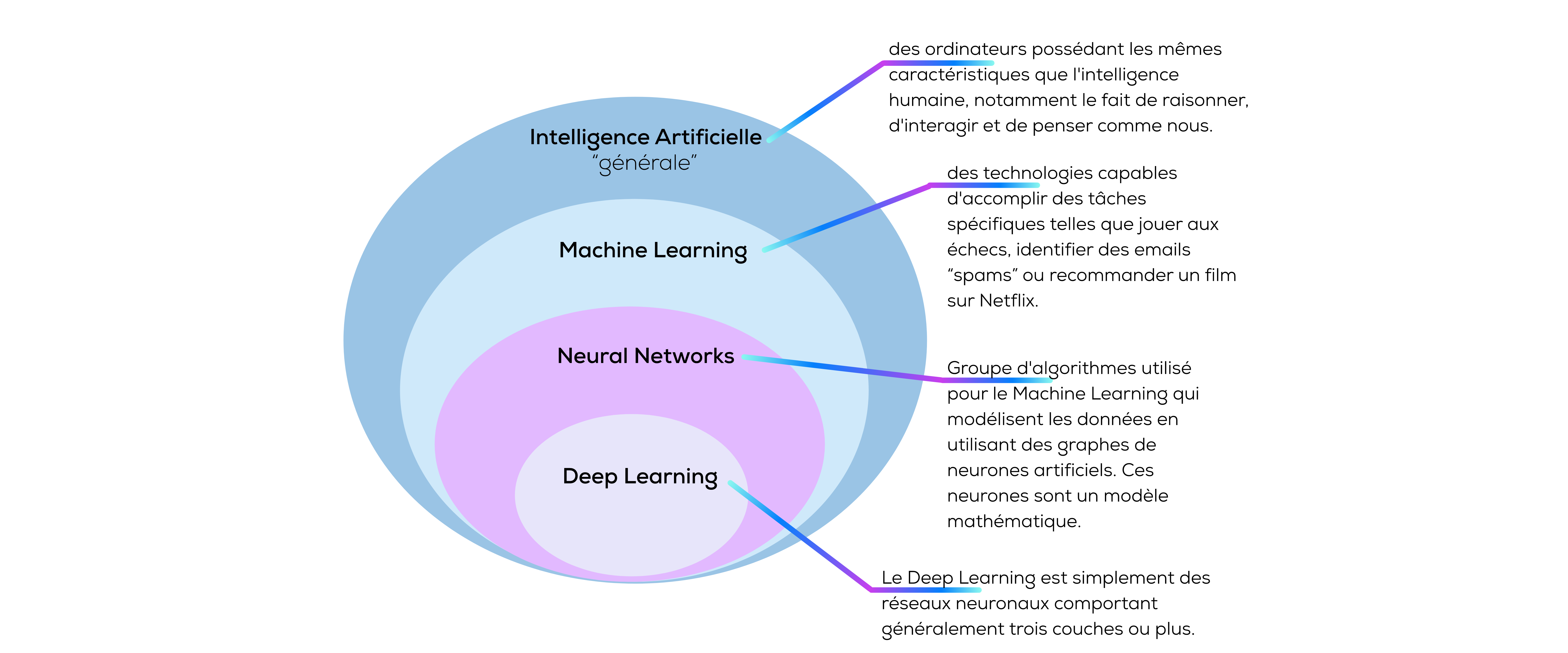
Artificial intelligence is a program or algorithm that uses a data set to recognize certain patterns. This allows it to draw conclusions or make predictions when it has sufficient information. It is composed of different subsets that can work together depending on the expected use.
In healthcare, the most widespread use of AI is "Machine Learning" because of its accuracy. Being able to predict which treatment procedures are likely to succeed in patients, based on their constitution and treatment setting, is a huge step forward for many healthcare organizations.
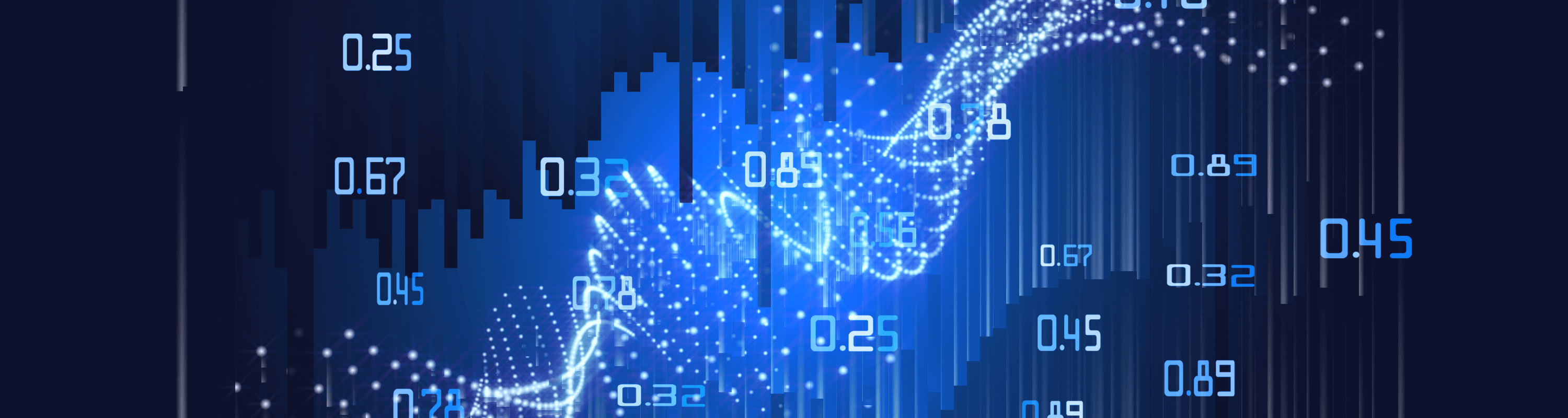
Types of artificial intelligence
Natural language programming
Most NLP (natural language processing) systems are forms of speech recognition, text analysis or translation. Making sense of human language has been a goal of artificial intelligence and healthcare technologies for over 50 years. One of the common uses of artificial intelligence in healthcare involves NLP applications that can understand and classify clinical documentation. NLP systems can also analyze unstructured clinical notes from patients. This provides valuable insight for understanding quality, improving methods, and, most importantly, better patient outcomes.
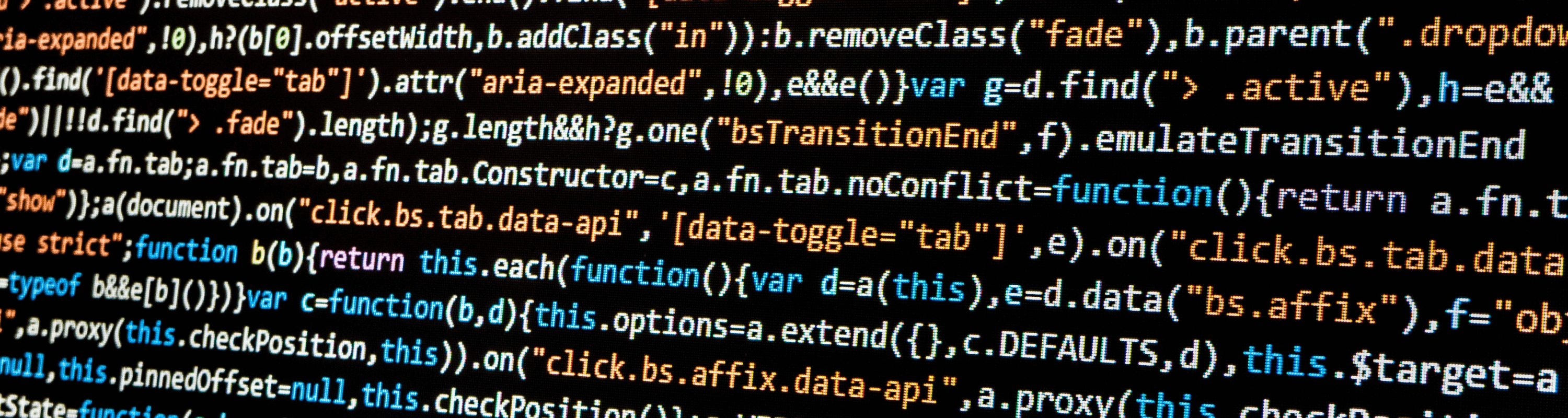
Rule-based expert systems
Expert systems based on "if-then" rule variations were the predominant AI technology in healthcare during the 1980s and beyond. In healthcare, this method is still widely used today for clinical decision support. Many electronic medical record (EMR) systems currently offer a set of rules with their software offerings.
Expert systems typically use human experts and engineers to construct an extensive set of rules in a certain knowledge domain. They work well up to a point and are easy to follow and process. However, when the number of rules becomes too large, usually exceeding several thousand, the rules can conflict with each other and cause the system to collapse. In addition, if the knowledge domain changes significantly, modifying the rules can be cumbersome and time consuming.
Computer vision
Computer vision is concerned with the recognition of visual elements. To teach a computer to understand visual data, it must be provided with a very large number of labeled images and then subjected to various software techniques or algorithms. This allows the computer to search for patterns and recognize them in all the elements related to these labels.
Among the most well-known innovations, we can mention facial recognition on our phones. We can also mention autonomous cars. Computer vision allows them to understand their environment in real time in order to identify roadsides, read traffic signs, detect other vehicles, objects and pedestrians.
In the healthcare field, computer vision is often used to look for symptoms on X-rays and MRIs.
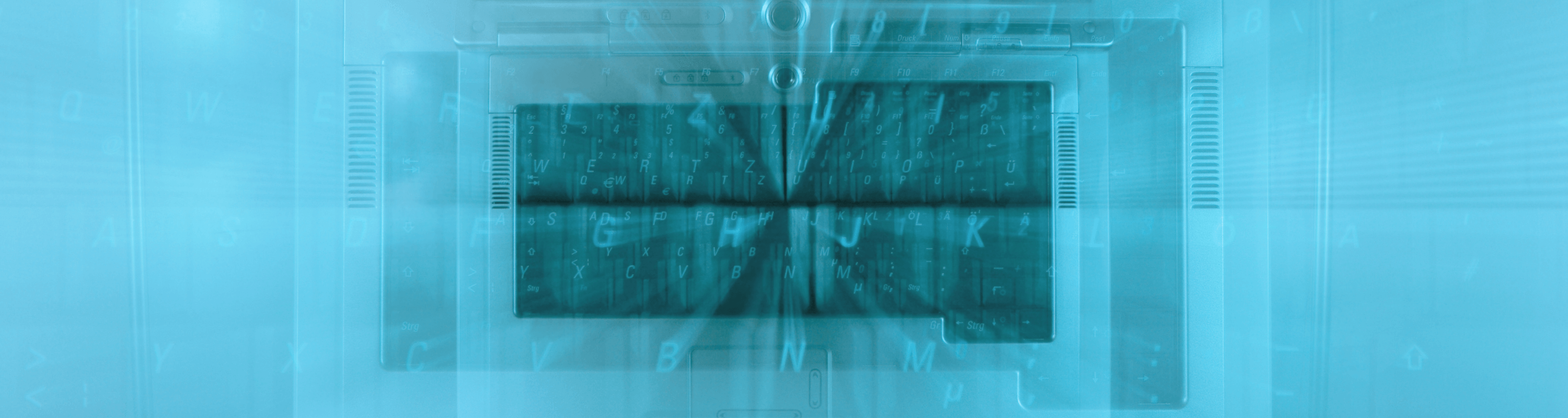
Audio processing
Audio processing involves taking real-world signals, such as voice, audio, video, temperature, pressure, or position that have been digitized, manipulating them mathematically to analyze them and lead to an action or decision. Apple's Siri, Amazon's Alexa and Google Assistant are the most popular examples of AI currently in use by the general public.
In sum, general AI is closer to science fiction movies, in which sentient machines mimic human intelligence. They are capable of strategic, abstract, and creative thinking, while managing a large number of complex tasks. Although machines can handle some tasks more efficiently than humans (such as data processing), this vision of a fully accomplished general AI is still only a fiction. This is why Human + Algorithm collaboration is essential. In the world as we know it today, artificial intelligence remains an extension of human capabilities. It does not replace them.
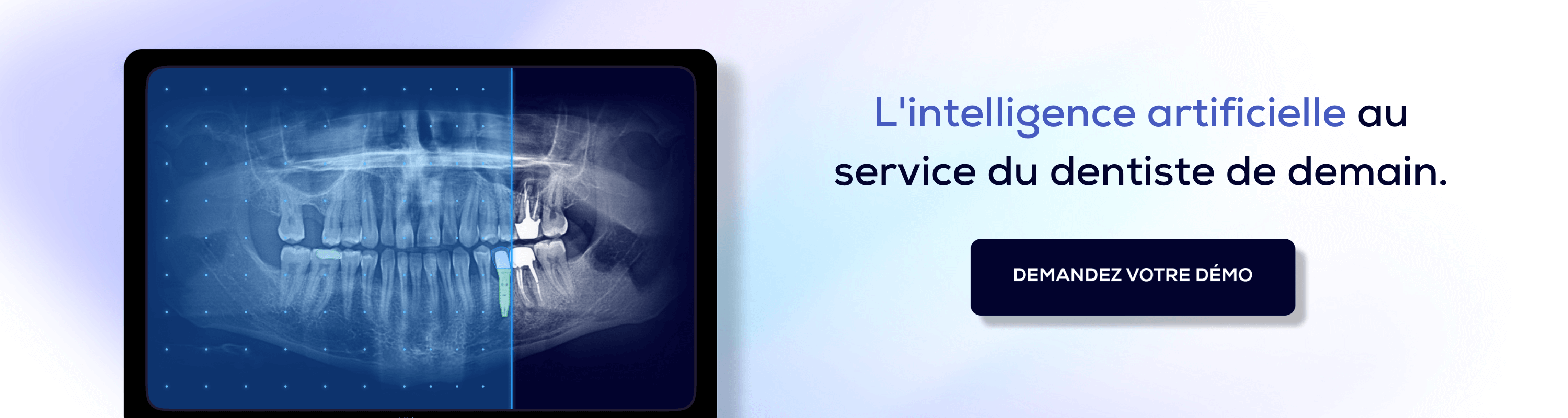
Artificial intelligence applications in healthcare
Artificial intelligence (AI) technologies, which are increasingly present in modern businesses and everyday life, are also constantly being applied to healthcare. Integrating AI into the healthcare ecosystem offers a multitude of benefits, including automating tasks and analyzing large patient datasets to provide better healthcare faster and at lower cost. According to Insider Intelligence, 30% of healthcare costs are related to administrative tasks. AI can automate some of these tasks, such as insurance pre-authorization, tracking unpaid bills, and record keeping, to ease the workload of healthcare professionals and ultimately save money.
AI also offers the potential to optimize diagnostic and prognostic pathways, and develop personalized treatment strategies through the use of large data sets. AI has the ability to analyze large data sets, gather patient information, and conduct predictive analysis. Obtaining patient information quickly helps the healthcare ecosystem discover key areas of patient care that need improvement.
Wearable healthcare technology is also using AI to better serve patients. Software that uses AI, such as FitBits and smartwatches, can analyze data to alert users and their healthcare professionals to potential health problems and risks. Being able to assess one's own health through technology eases the workload of professionals and prevents unnecessary hospital visits or readmissions.
Most AI and healthcare technologies have strong relevance to the healthcare field, but the tactics they support can vary widely between hospitals and other healthcare organizations. And while some articles on AI in healthcare suggest that the use of AI in healthcare can perform as well as or better than humans for some procedures, such as diagnosing diseases, it will be many years before AI in healthcare replaces humans for a wide range of medical tasks.
Let's take a look at some of the use cases for artificial intelligence and the benefits the healthcare industry can gain from their use.
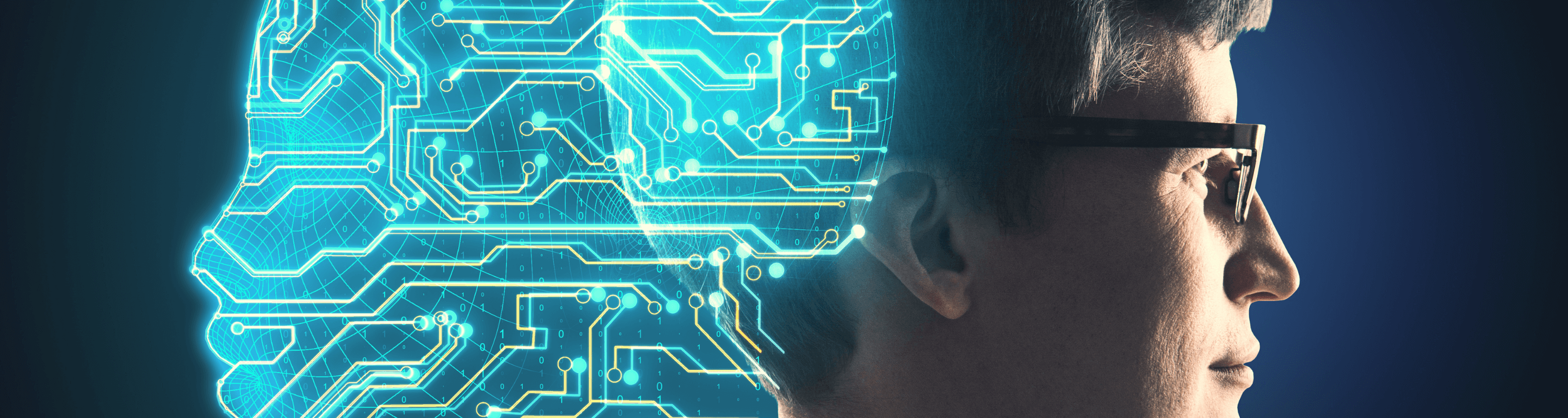
AI for early detection of skin cancer
Melanoma is the skin cancer with the poorest prognosis. If diagnosed at an early stage, it can be successfully treated with surgical procedures. However, once it reaches the metastatic stage, survival rates are significantly reduced. Visual inspection may not be sufficient to differentiate between benign and tumorous lesions. Diagnosis of melanoma depends on clinical examination and conventional biopsy findings of the lesion. The invasive nature of the procedure, the associated pain, and the need for repeated sampling for suspicious lesions with varied presentations are some of the limitations of skin biopsy.
Specialists can accurately diagnose cancer, however, given their limited numbers, there is a need to develop automated systems. AI can help in the early detection of skin cancer, thereby reducing the burden of disease and mortality associated with this disease. In addition to reducing the workload, AI-based systems can also help improve the diagnosis of skin lesions.
The International Skin Imaging Collaboration (ISIC): Melanoma Project is an international partnership between academia and industry to develop and promote standards for digital skin imaging to help reduce melanoma mortality. To this end, the ISIC proposes a collaboration that makes available open source dermoscopy images (+100,000 at present) and proposes competitions on artificial intelligence applied to dermatology (cancer detection, etc.).
In 2016, Codella et al. used the International Skin Imaging Collaboration dataset to compare the model to the performance of eight dermatologists in appraising 100 skin lesions as benign or malignant. Their AI models outperformed the dermatologists, with 76% accuracy and 62% specificity, compared with 70.5% accuracy and 59% specificity for the dermatologists.
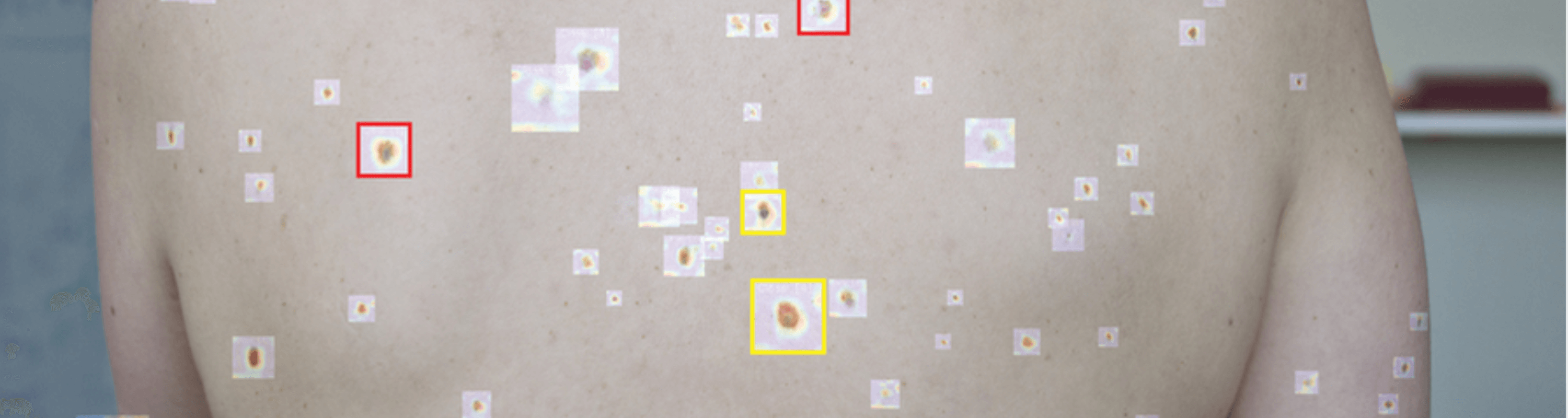
AI for early detection of Alzheimer's disease
It is not always clear when a person is in the early stages of Alzheimer's disease. Over time, in the background, the brain undergoes changes that can trigger subtle shifts in behavior and sleep patterns, many years before people show the obvious signs of dementia, such as confusion and memory loss. The researchers believe that artificial intelligence is capable of recognizing these changes at an early stage and identifying patients at risk of developing the worst forms of the disease.
A recent study found that artificial intelligence (AI) can detect Alzheimer's disease six years earlier than doctors. This is a significant advance in the fight against this disease that affects 5.7 million adults. This new AI algorithm, developed by the researchers, is able to detect signs of cognitive decline in brain MRIs, genetics and clinical data. This evidence can be used to predict whether the results will lead to Alzheimer's disease several years before symptoms appear.
A multidisciplinary research team involving several groups of researchers (Aramis project, Inserm & Cegedim) published, in April 2022, the results of research using a large database to identify risk factors for dementia due to Alzheimer's disease. This study is based on the analysis of the medical records of nearly 80,000 patients consulting general practitioners in France and the United Kingdom and on 123 possible data associations. These large data sets are analyzed from a mathematical and statistical point of view as well as from a medical and epidemiological point of view.
This analysis shows a list of 10 conditions frequently encountered in the 15 years before the onset of Alzheimer's disease. Among these 10 pathologies are depression, anxiety, exposure to high stress or hearing loss. Early detection by artificial intelligence systems allows the disease to be addressed at an early stage or even delayed.
The study will continue with the addition of over 26 million medical history data from Sweden and Australia. The study spectrum may be extended to other degenerative diseases (Parkinson's, Charcot disease, multiple sclerosis...).
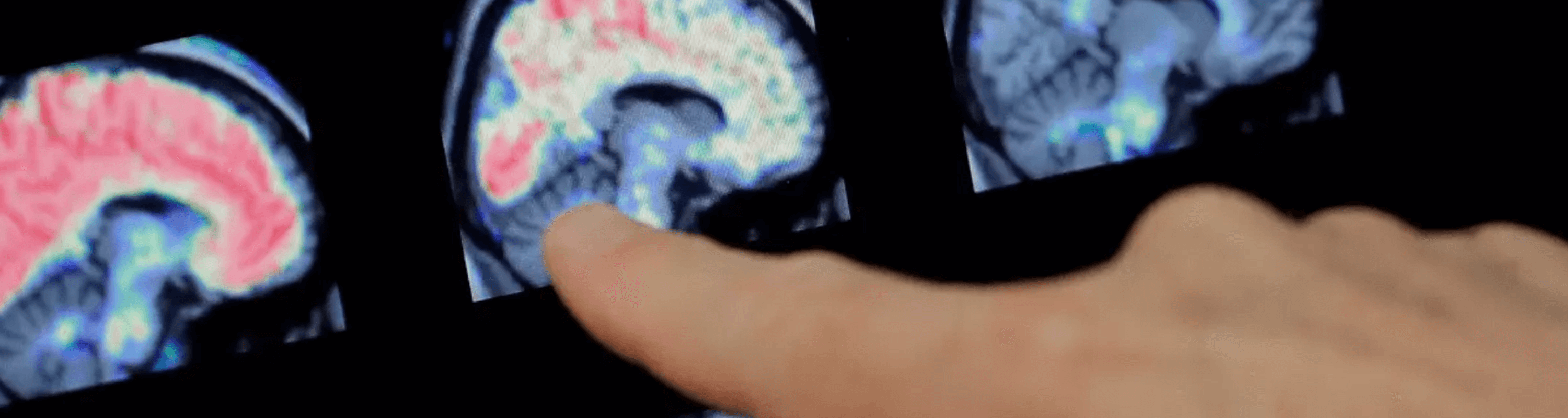
AI for early detection of breast cancer
As with melanoma, breast cancer can be successfully treated if diagnosed at an early stage. The goal of AI in this field is to detect lesions early and predict who might get them in the future. AI has demonstrated impressive accuracy and sensitivity in identifying imaging abnormalities, promising to improve tissue detection and characterization. For example, an analysis of screening mammograms showed that artificial neural networks are no more accurate than radiologists at detecting cancer, but they have consistently higher sensitivity for pathological findings, particularly for subtle lesions.
Two years ago, a team of scientists from MIT's Computer Science and Artificial Intelligence Laboratory (CSAIL) and the Jameel Clinic presented a deep learning system for predicting cancer risk from a single mammogram of a patient. The model showed promise, but the algorithms could be further improved. To do so, they adapted their new "Mirai" algorithm to the specific requirements of risk modeling. Mirai jointly models a patient's risk at multiple points in time and can potentially leverage clinical risk factors such as age or family history, if available. The algorithm is also designed to produce consistent predictions despite minor variations in clinical environments, such as choice of mammography device.
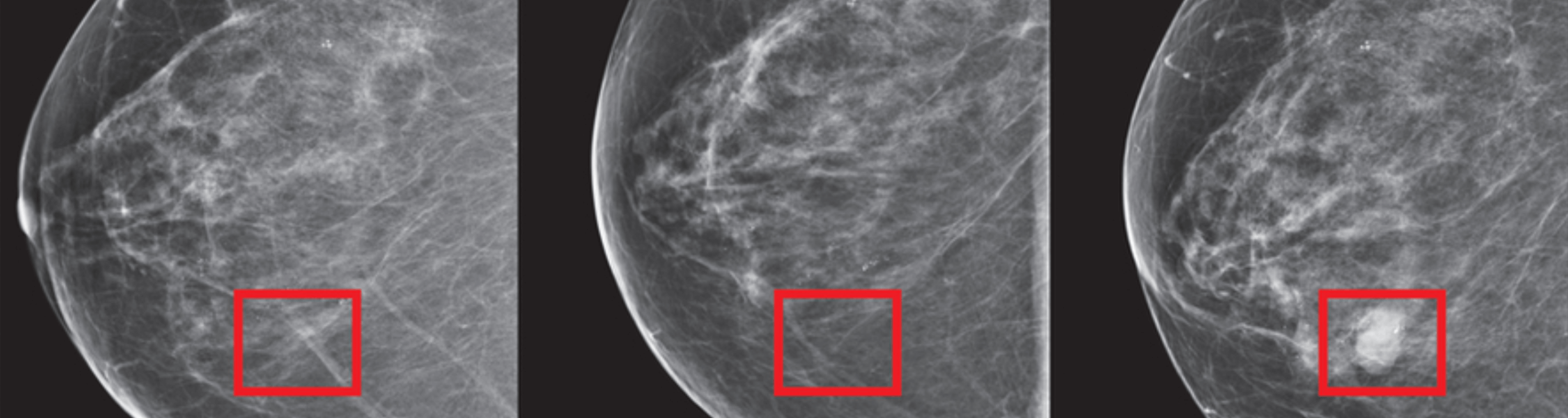
AI to improve administrative tasks
There are a number of administrative applications of artificial intelligence in healthcare. The use of AI in hospitals may be less revolutionary in the administrative aspects than in patient care. Nevertheless, artificial intelligence applied to hospital administrative management can lead to significant improvements in efficiency.
AI to reduce "no-shows
On average, a hospital experiences 12% no-shows daily. Out of 300 expected patients, that means 36 no-shows, and the staff is working at 88% capacity. At this rate, a hospital is losing about $5,400 per day, or $1.36 million per year, not including salary and infrastructure costs. AI would be able to assess the likelihood of a patient's no-show through a scoring system and apply a predictive analytics methodology to determine no-shows in real time by cross-referencing various data. Thus, it would be possible to follow up with the affected patients and offer other available slots.
AI in the service of cybersecurity
While cybersecurity is not strictly in the realm of operations, it remains one of the primary applications of AI in healthcare. AI-based solutions can help healthcare systems reduce risk by identifying unwanted behavior. They run constantly in the background to monitor activity and can even automatically add additional layers of security when a potential threat is detected.
AI at the service of management
AI in healthcare can combine historical hospital data, patient information, the current state of internal resources, external factors such as weather or disease outbreaks, and even behavioral science to optimize patient flow and staff resources. With the help of AI, these more accurate models of patient demand and bed utilization, powered by AI, can reduce average length of stay, dispatch ambulances more efficiently, prevent delayed discharges, and decrease patient wait times.
AI for supply chain management
The average annual procurement spend reduction opportunity for individual hospitals was $12.1 million in 2019. AI can analyze millions of data points on current inventory, past usage trends, and projected future usage to provide insights and recommendations for reducing procurement costs. These solutions can optimize inventory levels, increase standardization, improve workflow efficiency and supply throughput. AI can even alert staff to expired and recalled supplies. Today, artificial intelligence can bring many benefits to the healthcare industry, whether its application is medical or operational. The biggest challenge for AI in healthcare is not whether the technologies will perform well enough to be useful, but rather ensuring their adoption into everyday clinical practice. Over time, clinicians may migrate to tasks that require unique human skills, tasks that require the highest level of cognitive function. The only healthcare providers who may lose the full potential of AI in healthcare may be those who refuse to work alongside it.
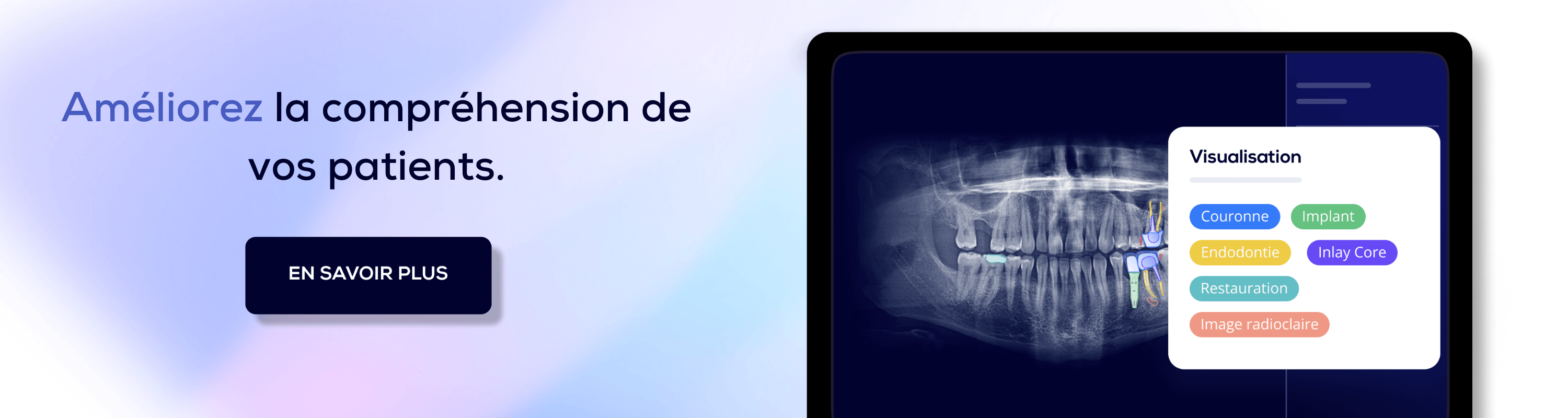
What about AI in dental practice?
Just as in general health, artificial intelligence in dentistry offers several axes:
- Clinical assistance through personalized, preventive and predictive diagnostic assistance thanks to its capacity to compare thousands of images in parallel and to analyze cases of evolution.
- A communication medium that contributes to patient education and oral prevention.
- Operational and administrative assistance thanks to its ability to automate a certain number of time-consuming tasks to which the dental surgeon does not bring any real added value.
Let's now discover the different typical cases of AI in dentistry.
Improving the detection of carious lesions
Dentists have always detected cavities, but the unanimity of reading an x-ray is far from 100%. A study asked three human dentists to examine and identify carious lesions on more than 8,000 x-rays to see how often dentists agree on the presence or absence of cavities. Dentists were unanimous in their opinion that 79% of the cases were caries-free. On the other hand, they were unanimous on the presence of cavities in only 4.2% of cases.
Computer vision systems can detect dental caries using techniques such as object detection and instance segmentation. One method is to train AI models on large sets of images with labeled carious lesions. Once the model training is complete, the algorithms are ready to receive raw data to identify these lesions on their own.
In this case, AI is used to provide a second opinion. It does not replace the expertise of dentists or the clinical examination, which is unavoidable, but instead helps them identify problems and potential treatments even more quickly and accurately. This saves time for the dentist, increases diagnostic accuracy and leaves more resources available for patient care.
Facilitate the treatment of periodontal disease
Periodontal diseases are the most common diseases in dentistry due to poor oral hygiene. Dentists are therefore able to detect these diseases, including the recession of the periodontal line and bone loss that can lead to tooth loss. All dentists are trained to determine the degree of disease through deep probing. However, it can be difficult to obtain an accurate history of a patient as well as accurate bone level measurements.
Artificial intelligence systems can quickly analyze the level of the periodontal line and highlight areas where the patient has bone level loss from an x-ray. This provides a more complete view of the patient's medical history. In addition, this technology is able to take measurements accurate to the millimeter, allowing the practitioner to quickly identify the treatment to be implemented.
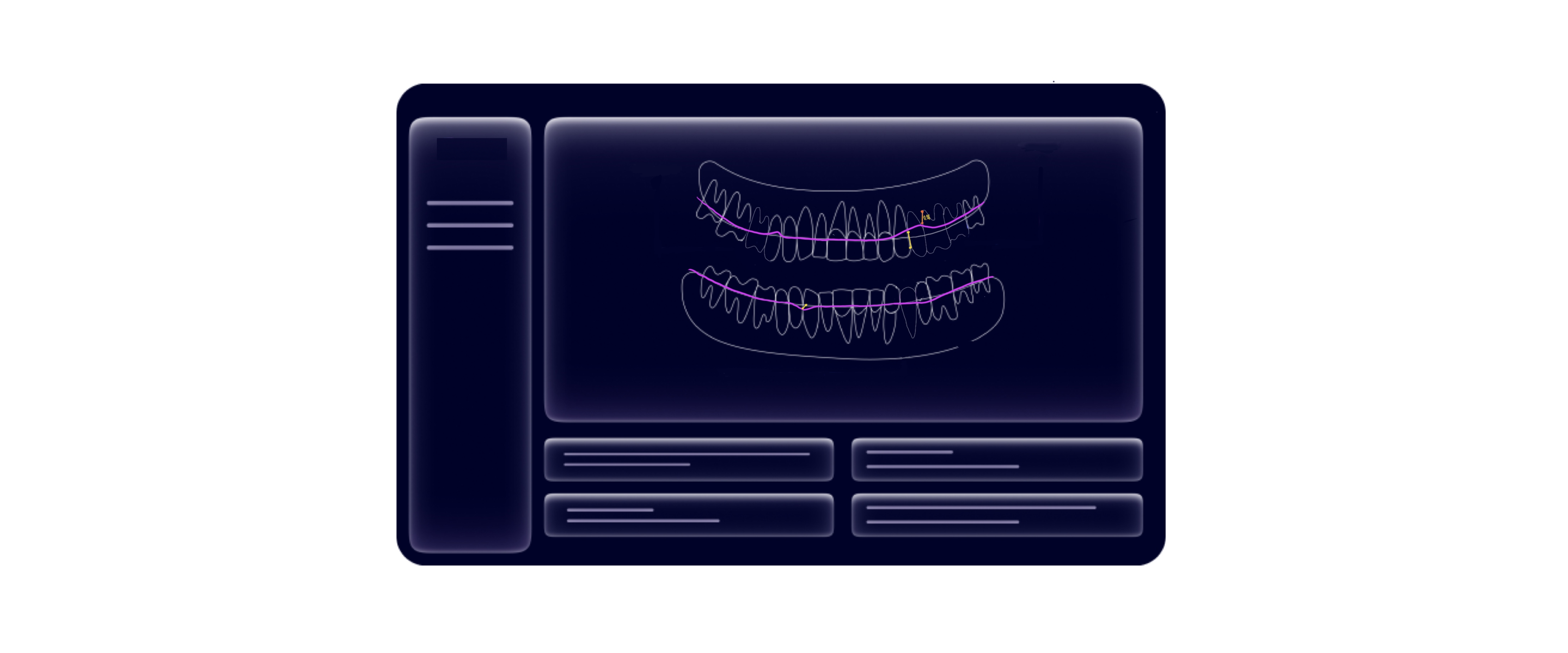
To facilitate the preparatory work for endodontic treatment
Endodontists typically use radiographic images to examine, measure, and evaluate the condition of the tooth in the gingiva down to the root. While detecting lesions in the tissues surrounding the root is important, determining the length of the root in the gum is also crucial to endodontic treatment.
Artificial intelligence models can also examine these images and determine structure, measurements, tissue viability, and even the potential for treatment success for parts of the tooth that are not visible. There are several methods to do this, including simply palpating the gum tissue, but reading dental x-rays is the most common. Deep learning algorithms can then detect, locate and classify different aspects of the tooth root anatomy as well as possible pathologies. This is useful for locating specific tooth structures or identifying particular types of lesions in or around the tooth.
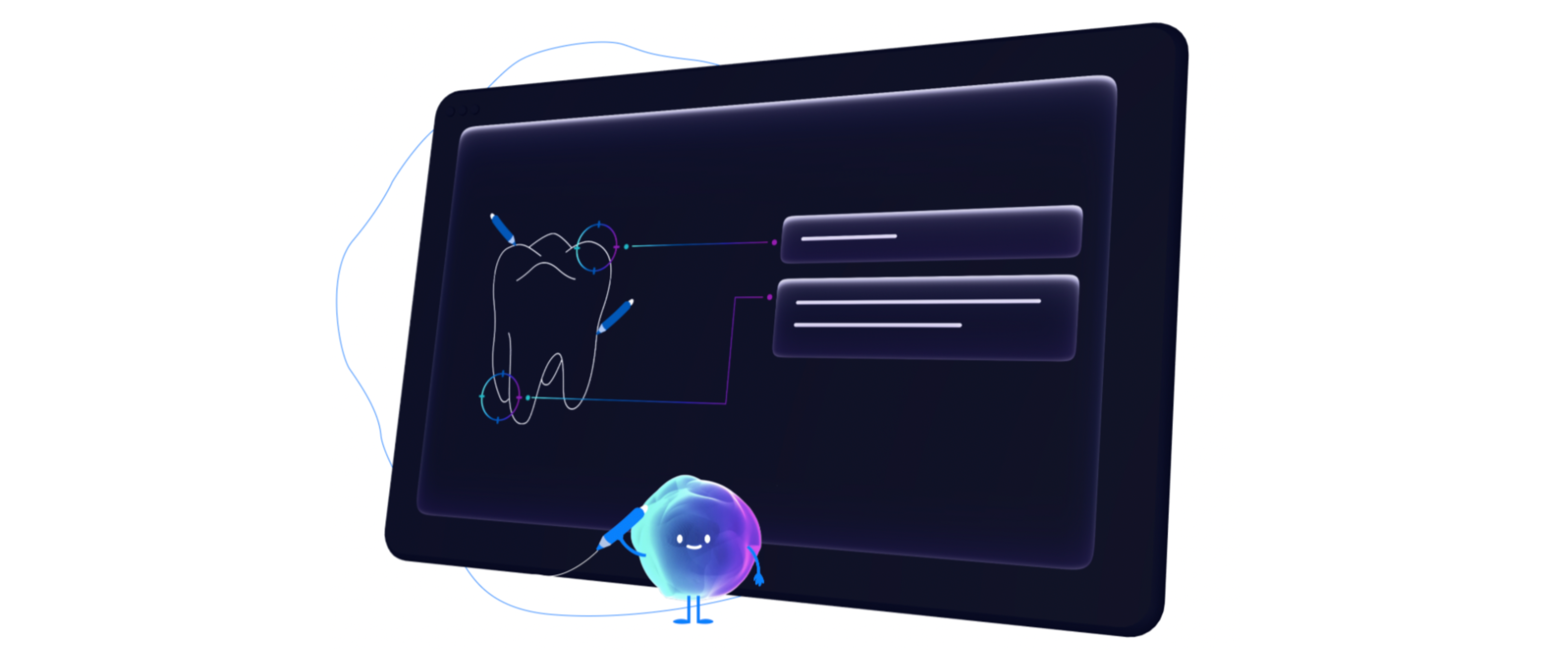
Facilitate the identification and placement of dental implants
Restoring an implant is a common task that can be a real headache when we have not placed the implant ourselves and we do not know its reference. In this case, the practitioner may have to do some tedious research to find the fitting and place the order.
However, computer vision technologies can identify implants and quickly obtain their references and connector size by comparing thousands of images.
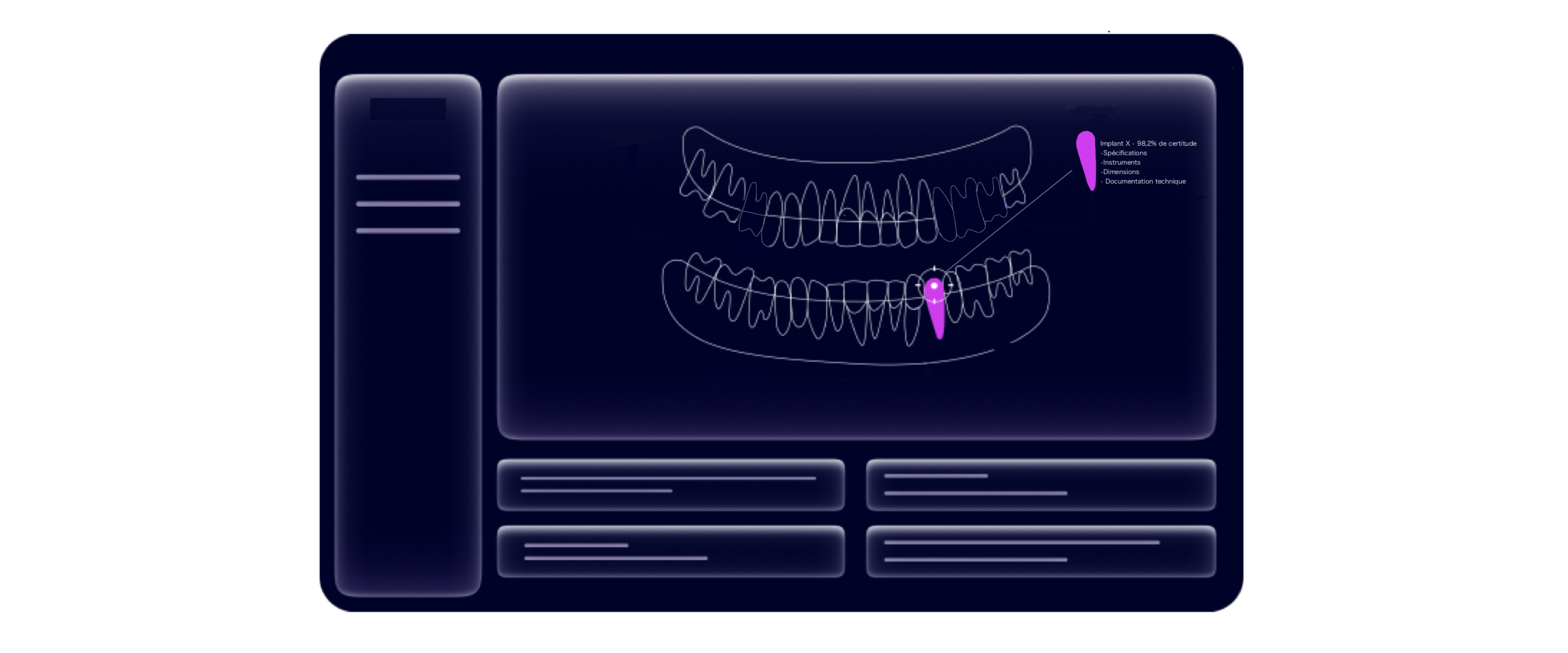
Assist in orthodontic treatment planning
Orthodontics requires extensive planning to determine the proper placement of each tooth, which teeth to extract and the best path for the teeth to move. Whether correcting a slight malocclusion or relieving an arthritic jaw, artificial intelligence is proving to be a valuable ally for dentists and orthodontists.
In this case, AI can be used to optimize analyses to predict things like the size of teeth that have not yet come out or the potential need for extraction. AI algorithms can also take a target starting point and end point, and then calculate the best way for a tooth or group of teeth to reach their optimal destination.
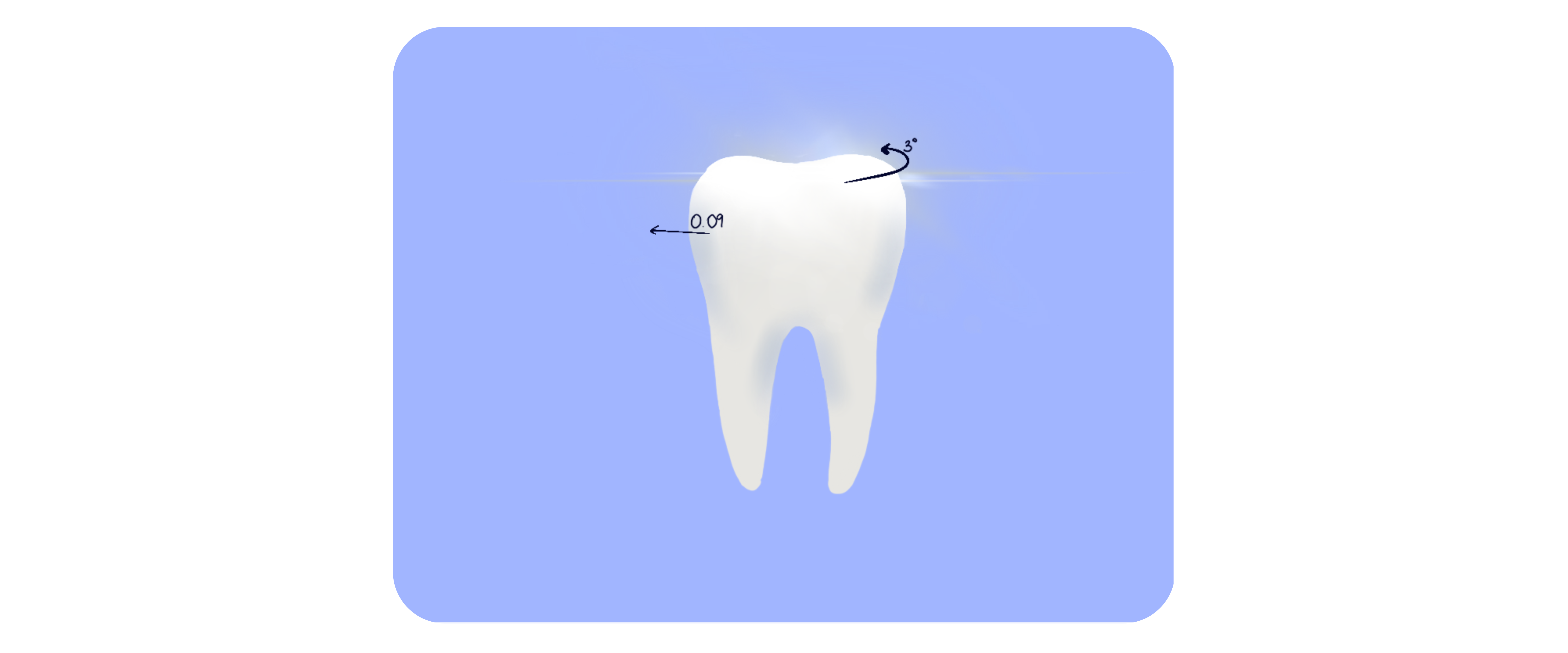
Facilitating patient understanding to further engage them in their oral health
Some of the key figures from our study on communication and patient satisfaction in the dental practice:
- 67% of respondents have difficulty locating their pathologies on their dental X-rays
- 63% of respondents feel that their patrician provides little or no explanation of their dental x-ray
- 34% of respondents think that a color-coded dental X-ray could help them identify pathologies more easily
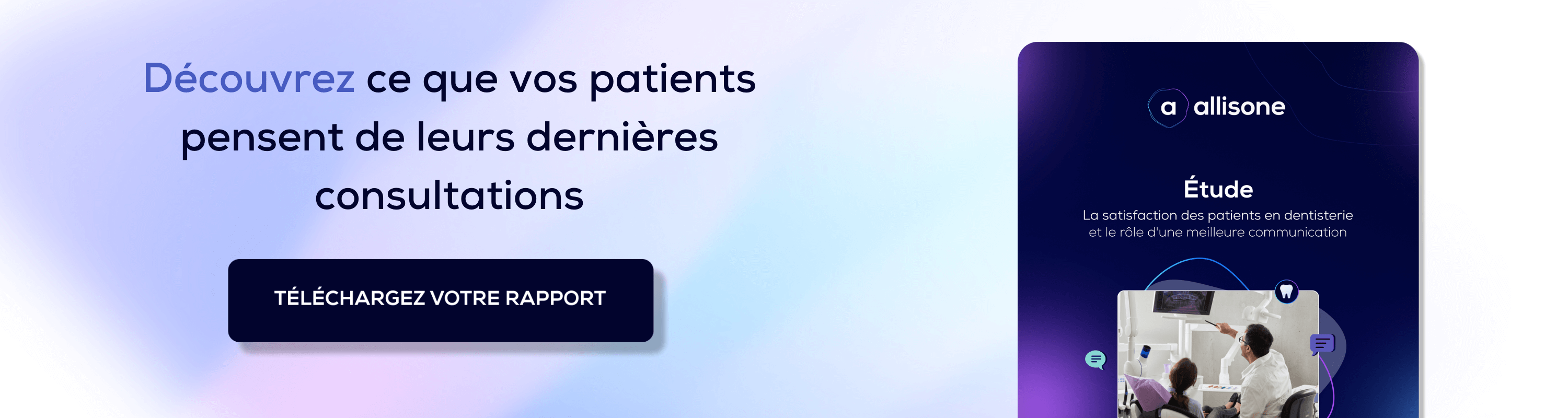
Although France is not yet ready to integrate Yomi, the Chinese robot capable of fitting two implants on a patient in just one hour, increasingly powerful tools are already providing many services to professionals. From intra-oral cameras to 3D impression software, digital tools are revolutionizing patient care overall: they improve the patient experience and optimize the performance of certain curative treatments, such as crowns or inlay-onlay. Although panoramic X-rays have been used for years to reassure patients by showing them what is actually happening in their mouths, for the layman, this black and white visual aid is essentially made up of shadowy areas that are difficult to interpret. And, sometimes, the dentist's explanations can seem rather vague and technical. Here, artificial intelligence can help you make your explanations more accessible.
This reading of panoramic radiographs, a key moment in the consultation and the patient's experience, is now greatly facilitated by the most advanced digital tools such asAllisone. The artificial intelligence ofAllisone allows the practitioner to highlight the elements on the X-ray thanks to a color code. The software facilitates patient understanding and simplifies chairside teaching. Thanks to this clear vision of the state of the mouth on a familiar digital interface (the screen), the patient is reassured and, above all, informed in a complete, adapted, transparent and playful way.
Post-consultation communication to build patient loyalty
Thanks to new technologies, the influence and experience with your dentist is no longer limited to the time of the office consultation. Sending e-mails summarizing the diagnosis and treatment plan has several advantages: it consolidates the practitioner-patient relationship, builds patient loyalty and promotes good oral health care in the short, medium and long term.
Of course, all this additional work brought by new technologies is time-consuming: practitioners and assistants rarely have, between two consultations or at the end of the day, the time necessary to send this type of message. This is where the most advanced technologies come into play: the SaaS software fromAllisone (accessible at any time from any terminal connected to the Internet) allows you to automate this crucial and time-consuming task. In just a few clicks, you create your treatment plan in Allisone and the solution takes care of communicating to your patient all the elements he needs to make the most appropriate decision: educational sheets, reports, illustrations of care, etc.
Overall, and even if it may seem paradoxical to the most digital-averse practitioners, new technologies make dental practice more human. They allow for better communication between patients and health professionals, facilitate the understanding of treatments and contribute to the improvement of the overall patient experience. As a result, patients are more likely to care about their oral health and adhere to proposed treatments. In this way, digital tools and artificial intelligence not only contribute to the effectiveness and accuracy of treatments, but also strengthen the relationship between patients and practitioners.
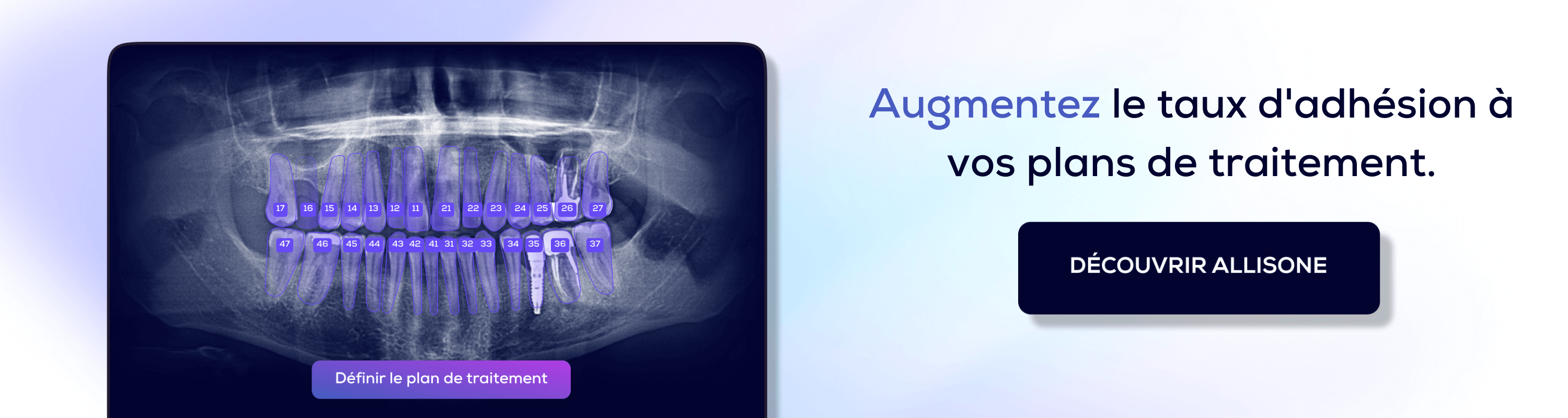
Simplify business processes
In addition to chairside work, dentists must perform a multitude of tasks such as creating treatment plans, preparing estimates, following up on patients and, of course, fulfilling the regulatory obligation to provide a report to their patients. IT, and more specifically automation and artificial intelligence technologies, can provide each practitioner with more comfort in performing these tasks and save time.
Here, AI may be able to identify lesions, suggest appropriate treatment plans and automate reporting. The technology can also centralize operational tasks within the practice, such as tracking treatment plans, reminders, appointment scheduling, etc.
In addition to working in the chair, the dentist performs a multitude of tasks such as creating treatment plans, drawing up estimates, following up on patients and, of course, fulfilling the regulatory obligation to provide a report to his patients. Information technology, and even more so automation and artificial intelligence technologies, can provide each practitioner with a little more comfort in these tasks and require less time.
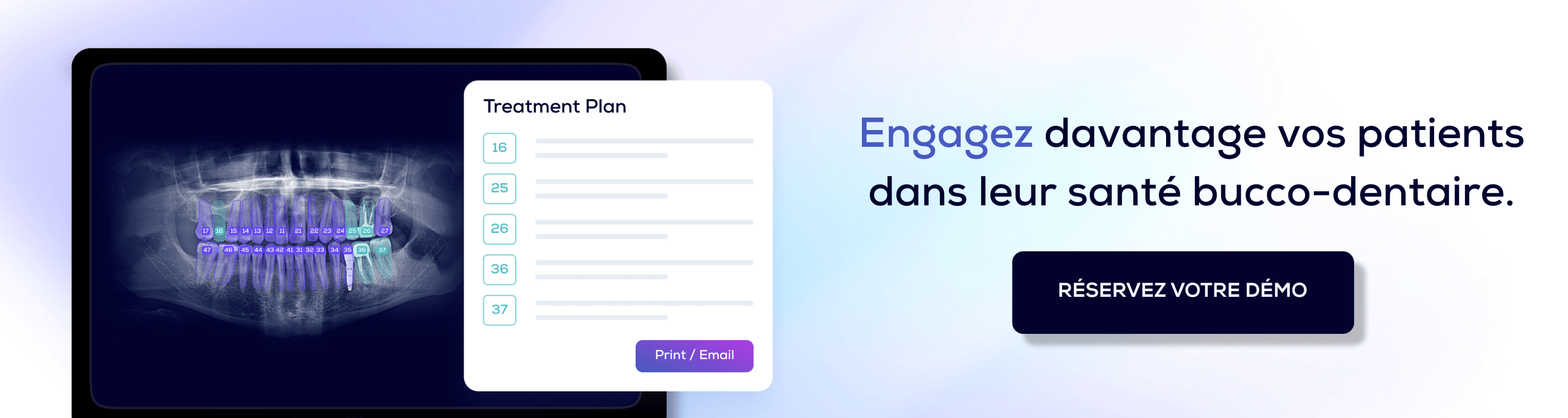